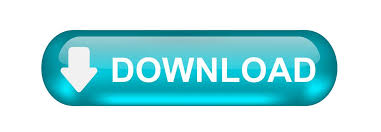
- #PRENTICE HALL GEOMETRY PRESENTATION ASSISTANT PLUS PDF#
- #PRENTICE HALL GEOMETRY PRESENTATION ASSISTANT PLUS INSTALL#
Zisserman, Very Deep Convolutional Networks for Large-Scale Visual Recognition, 2014 K. Oquab et al., Learning and Transferring Mid-Level Image Representations using Convolutional Neural Networks, CVPR 2014. Fergus, Visualizing and Understanding Convolutional Networks, ECCV 2014 M. LeCun et al., Gradient-based learning applied to document recognition, Proc. BengioĬonvolutional neural networks for visual recognition I. The website also includes links to useful reading materials such as “Practical Recommendations for Gradient-Based Training of Deep Architectures” by Y.

For more details on neural networks you can watch the video lectures by Hugo Larochelle. Supervised learning and deep learning optimization and regularization for neural networks (A. nearest neighbors with automatic algorithm configuration, VISAPP'09 Sivic & Zisserman, Video Google: Efficient visual search of videos (chapter from this book ), Philbin et al., Object retrieval with large vocabularies and fast spatial matching, CVPR'07 Jegou et al., Improving bag-of-features for large scale image search, IJCV 2010 Jegou et al., Aggregating local image descriptors into compact codes, PAMI 2011 Iscen et al., Efficient Diffusion on Region Manifolds, CVPR 2017 Arandjelovic et al., NetVLAD: CNN architecture for weakly-supervised place recognition, PAMI 2018.
#PRENTICE HALL GEOMETRY PRESENTATION ASSISTANT PLUS PDF#
Szeliski ( pdf ), Sections 4.1.3 (feature matching) and 6.1 (feature-based alignment) Szeliski ( pdf ), Sections 4.1, 4.1.1 and 4.1.2 from Chapter 4: Feature detection and matching R. Mundy - Object recognition in the geometric era: A retrospective. Lowe, Distinctive image features from scale-invariant keypoints, IJCV 2004 Mikolajczyk & Schmid, Scale and affine invariant interest point detectors, IJCV 2004 D. Introduction Class logistics, assignments, final projects When you enter the building, tell the receptionist you are going for a seminar. Typically, these are one hour research talks given by visiting speakers. You are welcome to attend seminars in the Willow group. If a plagiarism is detected, the student will be reported to MVA.Ĭomputer vision and machine learning talks Any uncredited reuse of material (text, code, results) will be considered as plagiarism and will result in zero points for the assignment / final project. The assignments and final projects will be checked to contain original material. Both students are expected to present the project at the oral presentation and contribute equally to writing the report. The final project report needs to explicitly specify the contribution of each student. If working in a group, we expect a more substantial project, and an equal contribution from each student in the group. For the final project, you may work alone or in a group of maximum of 2 people. However, each student has to work out their assignment alone (including any coding, experiments or derivations) and submit their own report. Discussions are encouraged and are an essential component of the academic environment. You can discuss the assignments and final projects with other students in the class. The final project will represent 50% of the grade. For additional technical instructions on the assignments please follow this link. The supporting materials for the programming assignments and final projects will be in Python and make use of Jupyter notebooks. There will be three programming assignments representing 50% (10% + 20% + 20%) of the grade. This course presents the image, object, and scene models, as well as the methods and algorithms, used today to address this challenge.
#PRENTICE HALL GEOMETRY PRESENTATION ASSISTANT PLUS INSTALL#
Python tutorial materials : Install anaconda, jupyter, run a notebook in colab.Īutomated object recognition - and more generally scene analysis - from photographs and videos is the grand challenge of computer vision. To register, fill in the Python tutorial participation form by October 6.Ĭlass Moodle: enroll in the course, get news, submit assignments, access discussion forum. Sign up for the course with your school account in Moodle.

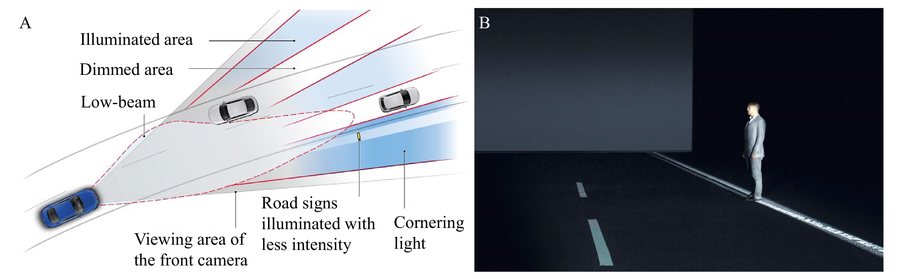
2022 Willow internship topics are out.Teaching assistants: Robin Strudel and Yann Labbé Lecture room: Salle Dussane, ENS Ulm, 45 rue d’Ulm, Paris (except Nov 16 and 23) Jean Ponce, Ivan Laptev, Cordelia Schmid and Josef Sivic Object recognition and computer vision 2021/2022 Reconnaissance d’objets et vision artificielle 2021/2022
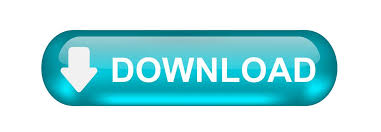